Try Blinkist to get the key ideas from 7,500+ bestselling nonfiction titles and podcasts. Listen or read in just 15 minutes.
Start your free trial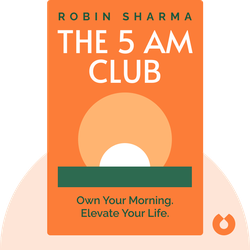
Blink 3 of 8 - The 5 AM Club
by Robin Sharma
Large-Scale Inference by Bradley Efron is a comprehensive guide to modern statistical methods for analyzing massive datasets. It covers techniques such as resampling, permutation tests, and model selection, providing valuable insights for researchers and practitioners.
In Large-Scale Inference, Bradley Efron begins by addressing the challenges faced in statistical inference when dealing with massive datasets. He introduces the reader to the concept of multiple hypothesis testing, a common scenario in modern scientific research, where thousands or even millions of hypotheses need to be tested simultaneously.
Efron explains how traditional inference methods, which were developed for small datasets, often fail to address the specific issues related to large-scale inference. He introduces the concept of the false discovery rate (FDR), which measures the proportion of false positives among the rejected hypotheses, and discusses how it has become a central concern in large-scale inference.
The author then delves into the Empirical Bayes approach, a statistical methodology that uses observed data to estimate the parameters of a prior distribution. He explains how this approach can be employed to address the multiple testing problem, providing a way to pool information across hypotheses and improve the accuracy of inference.
Efron illustrates the Empirical Bayes approach using various examples, including the estimation of the proportion of true null hypotheses, and the construction of confidence intervals for the FDR. He demonstrates how these methods can lead to more powerful and reliable inferences in large-scale settings.
Resampling methods, particularly the bootstrap, play a significant role in large-scale inference. Efron presents a detailed discussion on the bootstrap method and its applications in large-scale settings. He demonstrates how the bootstrap can be used to estimate the FDR, and how it can help in making accurate inferences when dealing with complex, high-dimensional data.
The author also discusses the limitations of the bootstrap and other resampling methods in large-scale inference, including their computational complexity and assumptions. He provides insights on when and how to use resampling methods effectively in the face of big data challenges.
Efron then turns his attention to Bayesian methods and their role in large-scale inference. He discusses the advantages of the Bayesian approach, such as its flexibility in handling complex models and its ability to provide probabilistic statements about the parameters of interest.
However, the author also highlights the challenges of applying Bayesian methods in large-scale settings, including the computational burden of sampling from high-dimensional posterior distributions. He presents recent developments, such as approximate Bayesian computation and variational inference, as potential solutions to these challenges.
In the final section of Large-Scale Inference, Efron looks ahead to the future of large-scale inference. He discusses recent advances, such as the use of deep learning in statistical inference and the development of scalable algorithms for high-dimensional problems.
The author also outlines several open problems in large-scale inference, including the development of more accurate FDR estimation methods and the integration of domain-specific knowledge into statistical models. He concludes by emphasizing the importance of continued research in this area, given the increasing prevalence of big data in scientific and technological domains.
Large-Scale Inference by Bradley Efron explores the challenges and opportunities presented by the vast amounts of data available in modern scientific research. The book delves into the methods and techniques used to draw meaningful conclusions from large datasets, covering topics such as multiple testing, resampling methods, and the role of computational power in statistical inference. It offers valuable insights for anyone working with big data in fields such as genetics, economics, and social sciences.
Statisticians and data scientists looking to understand and apply large-scale inference methods
Researchers and practitioners in fields such as genomics, neuroscience, and economics where massive datasets are common
Graduate students and academics interested in cutting-edge statistical methods and their practical implications
It's highly addictive to get core insights on personally relevant topics without repetition or triviality. Added to that the apps ability to suggest kindred interests opens up a foundation of knowledge.
Great app. Good selection of book summaries you can read or listen to while commuting. Instead of scrolling through your social media news feed, this is a much better way to spend your spare time in my opinion.
Life changing. The concept of being able to grasp a book's main point in such a short time truly opens multiple opportunities to grow every area of your life at a faster rate.
Great app. Addicting. Perfect for wait times, morning coffee, evening before bed. Extremely well written, thorough, easy to use.
Try Blinkist to get the key ideas from 7,500+ bestselling nonfiction titles and podcasts. Listen or read in just 15 minutes.
Start your free trialBlink 3 of 8 - The 5 AM Club
by Robin Sharma